Advantages and Disadvantages of Machine Learning
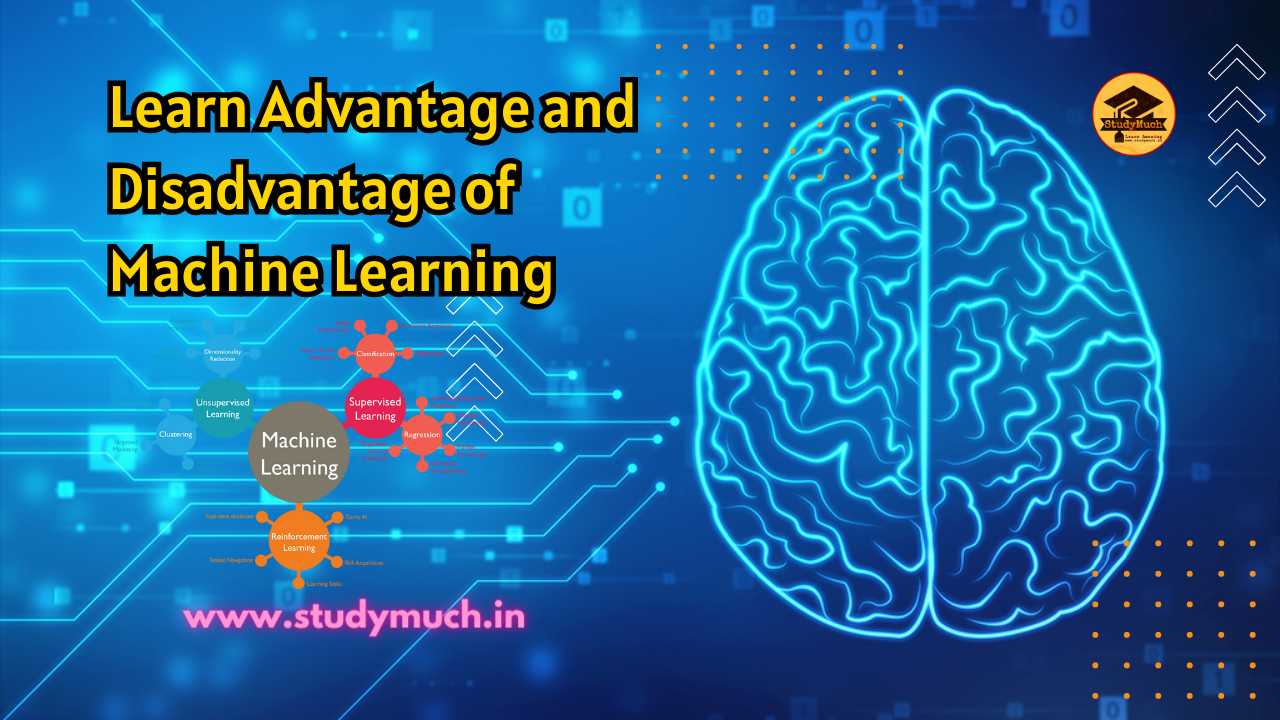
Advantages and Disadvantages of Machine Learning
Machine learning, a subset of artificial intelligence, has revolutionized numerous industries by empowering computers to learn from data and improve their performance without explicit programming. From self-driving cars to personalized recommendations, machine learning has become a crucial technology in today’s world. Like any other technology, machine learning comes with its own set of advantages and disadvantages. In this blog post, we will delve into the various aspects of machine learning, exploring its strengths and weaknesses, its Features and Uses, Advantages and Disadvantages of Machine Learning.
Features of Machine Learning:
- Automation: Machine learning enables automated decision-making and problem-solving without human intervention.
- Adaptability: ML models can learn and adapt from new data, improving their performance over time.
- Pattern Recognition: ML can identify complex patterns and trends in large datasets, making it useful for data analysis.
- Scalability: ML algorithms can handle large-scale datasets and computations efficiently.
- Prediction: ML models can predict outcomes and trends based on historical data and patterns.
- Personalization: ML enables personalized recommendations and experiences for users.
- Real-time Processing: Some ML algorithms can process data in real-time, allowing for immediate responses.
Advantages of Machine Learning:
Automation and Efficiency:
Machine learning enables automation of repetitive tasks, saving time and resources for businesses. It can handle vast amounts of data and perform complex computations at speeds unattainable by humans. This efficiency fosters productivity and frees up human resources for more creative and strategic tasks.
Decision Making and Predictive Analytics:
Machine learning models can analyze historical data to identify patterns and trends, making them valuable tools for decision-making and predictive analytics. Businesses can leverage this capability to optimize processes, improve customer experiences, and gain a competitive edge.
Personalization:
Machine learning allows businesses to offer personalized experiences to their customers. Recommendation systems in e-commerce and content platforms are prime examples of how machine learning can enhance user satisfaction by suggesting products or content tailored to individual preferences.
Handling Big Data:
With the exponential growth of data, traditional data analysis methods have become inadequate. Machine learning techniques can handle vast datasets and extract valuable insights, helping organizations make data-driven decisions.
Continuous Learning and Improvement:
Machine learning models can continuously learn from new data, adapting and improving over time. This adaptability is particularly valuable in dynamic environments, where changes and trends are frequent.
Medical Applications:
In the medical field, machine learning has the potential to revolutionize diagnostics and treatment. It can analyze medical images, predict disease outcomes, and assist in drug discovery, ultimately leading to improved patient care and outcomes.
Disadvantages of Machine Learning:
Here are some important disadvantages of Machine Learning;
Data Dependency:
The effectiveness of machine learning models heavily relies on the quality and quantity of data available for training. Insufficient or biased data can lead to inaccurate predictions or reinforce existing biases, which may result in unfair or discriminatory outcomes.
Overfitting:
Overfitting occurs when a machine learning model performs exceptionally well on the training data but fails to generalize to new, unseen data. It is a common challenge that can lead to unreliable predictions.
Interpretability and Transparency:
Complex machine learning models, such as deep neural networks, can be challenging to interpret. This lack of transparency raises concerns about the model’s decision-making process, especially in critical applications like healthcare and finance.
High Computational Resources:
Training sophisticated machine learning models can demand substantial computational power and memory. This requirement can be expensive and may limit the accessibility of certain machine learning techniques to smaller organizations or individuals.
Ethical and Security Concerns:
As machine learning models influence significant aspects of people’s lives, there are ethical considerations related to privacy, security, and data ownership. Ensuring fairness and avoiding misuse of AI-powered systems is a paramount challenge.
Limited Creativity and Common Sense:
Machine learning models excel in tasks for which they are trained but lack human-like creativity and common sense. They may struggle to handle novel situations or require extensive retraining to adapt to new scenarios.
Uses of Machine Learning:
- Natural Language Processing (NLP): ML is used for language translation, sentiment analysis, chatbots, etc.
- Image Recognition: ML is used in facial recognition, object detection, and image classification tasks.
- Recommendation Systems: ML powers recommendation engines for personalized product suggestions.
- Fraud Detection: ML can detect fraudulent activities in financial transactions.
- Medical Diagnosis: ML aids in diagnosing diseases and predicting patient outcomes.
- Autonomous Vehicles: ML is utilized in self-driving cars for navigation and decision-making.
- Financial Analysis: ML models can predict stock prices and analyze financial markets.
- Virtual Assistants: ML powers virtual assistants like Siri and Alexa for voice-based interactions.
- Speech Recognition: ML enables speech-to-text and voice commands in various applications.
- Email Spam Filtering: ML algorithms can identify and filter out spam emails.
These are just some of the many features and uses of machine learning, showcasing its versatility and impact across diverse industries and applications.
Conclusion:
Machine learning has transformed the way we interact with technology, offering a plethora of advantages across various industries. The ability to automate tasks, make data-driven decisions, and provide personalized experiences has significantly improved operational efficiency and customer satisfaction. However, machine learning is not without its challenges. Issues related to data quality, model interpretability, and ethical considerations must be addressed to harness its full potential responsibly.
As the field of machine learning continues to advance, striking a balance between its advantages and disadvantages will be critical in harnessing its power for the betterment of society. Emphasizing transparency, fairness, and ethical use of machine learning will pave the way for a future where AI technologies coexist harmoniously with human values and aspirations.
Learn More;
0 Comments